Introduction to Pandas
[3]:
import pandas as pd
import seaborn as sns
import matplotlib.pyplot as plt
from pprint import pprint
What is a pandas DataFrame
Pandas is a library
(import it, has a documentation website https://pandas.pydata.org/)
DataFrame is a datatype/datastructure/object
the main offering of the pandas library
Why use pandas DataFrames
Pandas DataFrame Description/Motivation
Robust tool for data wrangling and data analysis
Exceptionally good documentation
Synergizes with other libraries
More powerful than excel
Use-case
In-memory amount of data
Millions of rows
Clear example of DataFrame
Attributes
Column Indexes
Row Indexes
Multiple datatypes
Datatypes function of column
List of strings in the entry at [4,‘column_three’]
How are they made?
They are declared
The information comes from
read in a .csv file
from a python dictionary
from a pickle (special binary file)
from .json file
and more
Making the above DataFrame
Strategy
DataFrame from a python dictionary (less common, but helpful for now)
If we were unsure
We make a dictionary where
the keys are column names
the values are lists of actual data
[4]:
#make the values
first_list=[1,2,3,4,5]
second_list=['a','b','c','d','e']
third_list=['lets','mix','it','up',['ok']]
[5]:
#assign the values to keys in a dictionary
my_dict={
'column_one':first_list,
'column_two':second_list,
'column_three':third_list
}
[6]:
#print the whole dictionary to see what it looks like
#note that the keys are ordered alphabetically when we use pprint (pretty print)
pprint(my_dict)
{'column_one': [1, 2, 3, 4, 5],
'column_three': ['lets', 'mix', 'it', 'up', ['ok']],
'column_two': ['a', 'b', 'c', 'd', 'e']}
[7]:
#declare our dataframe using the "from dictionary approach"
#get our "information" from our dictionary
my_DataFrame=pd.DataFrame.from_dict(my_dict)
[8]:
#see our dataframe
my_DataFrame
[8]:
column_one | column_two | column_three | |
---|---|---|---|
0 | 1 | a | lets |
1 | 2 | b | mix |
2 | 3 | c | it |
3 | 4 | d | up |
4 | 5 | e | [ok] |
Accessing Values in a DataFrame
Accesing the Indices (indexes)
The indices are accessible and mutable (changeable)
[9]:
print(my_DataFrame.index)
RangeIndex(start=0, stop=5, step=1)
[10]:
print(my_DataFrame.columns)
Index(['column_one', 'column_two', 'column_three'], dtype='object')
Accesing cells by numeric location
Values DataFrames can be accessed “like” traditional lists (according to numerical position).
[11]:
my_DataFrame.iloc[2,1]
[11]:
'c'
This can be a mildly dangerous approach if you are writing for long-term projects. But can be convenient for some quick-scripts.
Accessing cells by index/column-names
“at” is a good choice for single-value access
[12]:
my_DataFrame.at[2,'column_three']
[12]:
'it'
“loc” is a good choice for “slicing” a dataframe (provide a list of row-indices and a list of column-indices)
[13]:
my_DataFrame.loc[0:2,['column_two','column_three']]
[13]:
column_two | column_three | |
---|---|---|
0 | a | lets |
1 | b | mix |
2 | c | it |
Accessing cells by condition
We can write conditions “inside loc” in order to get slices where the condition is true (fyi: under the hood, python turns the condition into a list of True/False)
[14]:
my_DataFrame.loc[
my_DataFrame['column_one'] > 2
]
[14]:
column_one | column_two | column_three | |
---|---|---|---|
2 | 3 | c | it |
3 | 4 | d | up |
4 | 5 | e | [ok] |
Operations on a DF
We can loosely classify operations on a DF into “simple” and “complicated”. The general strategy for each type of operation is shown below.
Simple Operations
Rule of thumb definition
If the operation feels “common”, then see (google) if there is a built-in function
Examples
Taking the average of a column
Adding a constant to a column
Stripping the whitespace from the ends of strings in a column
Advice
Use the built in function.
Fast
Error-free
[15]:
#taking the average value of a column
#same thing as my_DataFrame['column_one'].mean()
my_DataFrame.column_one.mean()
[15]:
3.0
[16]:
#adding a constant value to a column
my_DataFrame.column_one+5
[16]:
0 6
1 7
2 8
3 9
4 10
Name: column_one, dtype: int64
A Caveat
[17]:
#notice in the above we did not assign the output of "my_DataFrame.column_one+5" to anything.
#so the original dataframe remains unchanged
my_DataFrame
[17]:
column_one | column_two | column_three | |
---|---|---|---|
0 | 1 | a | lets |
1 | 2 | b | mix |
2 | 3 | c | it |
3 | 4 | d | up |
4 | 5 | e | [ok] |
[18]:
#removing the whitespace from a column
my_DataFrame.column_two.str.strip()
[18]:
0 a
1 b
2 c
3 d
4 e
Name: column_two, dtype: object
Notice that we needed to “access” the “string representation” of a column in order to “do an operation” that “acts on strings”. With a little practice you will get used to these things.
More Complicated Operations
Rule of thumb definition
As the “customness” of an operation increases, so do the chances that you will have to write the operation yourself.
Advice
If the project does not call for it, do not break your back to force the use of fast functions.
Instead, consider “operating” “element-wise” “in a for-loop”
Examples
Each element in a column is searched against a database
Each list in a column has some complicated math done on it
One approach is iterrows
[30]:
#iterrows gives us two things that we iterate over simultaneously, much like enumerate() on a
#"normal" list
for temporary_index,temporary_row in my_DataFrame.iterrows():
#COMPLICATED CODE HERE
#printing example:
if temporary_index==2:
print(temporary_index)
print('*'*30)
print(temporary_row)
print('*'*30)
print(temporary_row['column_two'])
2
******************************
column_one 3
column_two c
column_three it
Name: 2, dtype: object
******************************
c
Synergies with other libraries
Getting a larger dataset
[20]:
#the penguins dataset is a "classic"
my_dataset=sns.load_dataset('penguins')
[21]:
my_dataset
[21]:
species | island | bill_length_mm | bill_depth_mm | flipper_length_mm | body_mass_g | sex | |
---|---|---|---|---|---|---|---|
0 | Adelie | Torgersen | 39.1 | 18.7 | 181.0 | 3750.0 | Male |
1 | Adelie | Torgersen | 39.5 | 17.4 | 186.0 | 3800.0 | Female |
2 | Adelie | Torgersen | 40.3 | 18.0 | 195.0 | 3250.0 | Female |
3 | Adelie | Torgersen | NaN | NaN | NaN | NaN | NaN |
4 | Adelie | Torgersen | 36.7 | 19.3 | 193.0 | 3450.0 | Female |
... | ... | ... | ... | ... | ... | ... | ... |
339 | Gentoo | Biscoe | NaN | NaN | NaN | NaN | NaN |
340 | Gentoo | Biscoe | 46.8 | 14.3 | 215.0 | 4850.0 | Female |
341 | Gentoo | Biscoe | 50.4 | 15.7 | 222.0 | 5750.0 | Male |
342 | Gentoo | Biscoe | 45.2 | 14.8 | 212.0 | 5200.0 | Female |
343 | Gentoo | Biscoe | 49.9 | 16.1 | 213.0 | 5400.0 | Male |
344 rows × 7 columns
Note that we can no longer see every row and every column. This will be especially true in datasets with thousands (of thousands) of rows. We want to be able to interrogate the dataset as a whole.
Basic inspection - Some handy functions
[22]:
my_dataset.info()
<class 'pandas.core.frame.DataFrame'>
RangeIndex: 344 entries, 0 to 343
Data columns (total 7 columns):
# Column Non-Null Count Dtype
--- ------ -------------- -----
0 species 344 non-null object
1 island 344 non-null object
2 bill_length_mm 342 non-null float64
3 bill_depth_mm 342 non-null float64
4 flipper_length_mm 342 non-null float64
5 body_mass_g 342 non-null float64
6 sex 333 non-null object
dtypes: float64(4), object(3)
memory usage: 18.9+ KB
info() shows us
column names
non-null counts
datatypes
memory usage
[23]:
my_dataset.describe()
[23]:
bill_length_mm | bill_depth_mm | flipper_length_mm | body_mass_g | |
---|---|---|---|---|
count | 342.000000 | 342.000000 | 342.000000 | 342.000000 |
mean | 43.921930 | 17.151170 | 200.915205 | 4201.754386 |
std | 5.459584 | 1.974793 | 14.061714 | 801.954536 |
min | 32.100000 | 13.100000 | 172.000000 | 2700.000000 |
25% | 39.225000 | 15.600000 | 190.000000 | 3550.000000 |
50% | 44.450000 | 17.300000 | 197.000000 | 4050.000000 |
75% | 48.500000 | 18.700000 | 213.000000 | 4750.000000 |
max | 59.600000 | 21.500000 | 231.000000 | 6300.000000 |
describe() gives us some descriptive statistics of numeric columns
look at these values together (dont assume normality, etc)
Visualizing our Dataset
[24]:
#seaborn's pairplot very conveniently accepts a dataframe as input
#and makes a scatter/histogram figure
sns.pairplot(
my_dataset
)
[24]:
<seaborn.axisgrid.PairGrid at 0x7fdd79011f40>
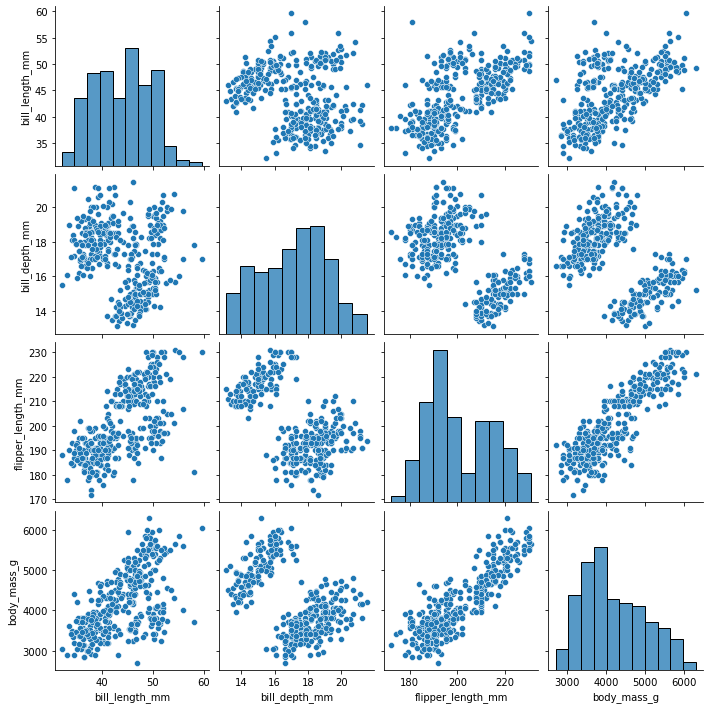
[25]:
#we could send a smaller dataframe using a list of columns if we wanted
sns.pairplot(
my_dataset[['bill_length_mm','bill_depth_mm']]
)
[25]:
<seaborn.axisgrid.PairGrid at 0x7fdd79121a00>
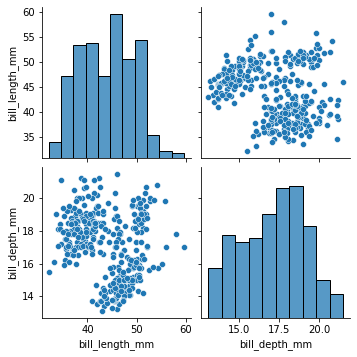
[26]:
sns.pairplot(
my_dataset,
#seaborn is built "on top of matplotlib", which means that it does a lot of things
#more easily for you
#however, some of the stuff in matplotlib is still accessible if you can express
#what you want the same way.
#if we had millions of datapoints, we could visualize density by making the points
#somewhat transparent
plot_kws={'alpha':0.1}
)
[26]:
<seaborn.axisgrid.PairGrid at 0x7fdd7529e1f0>
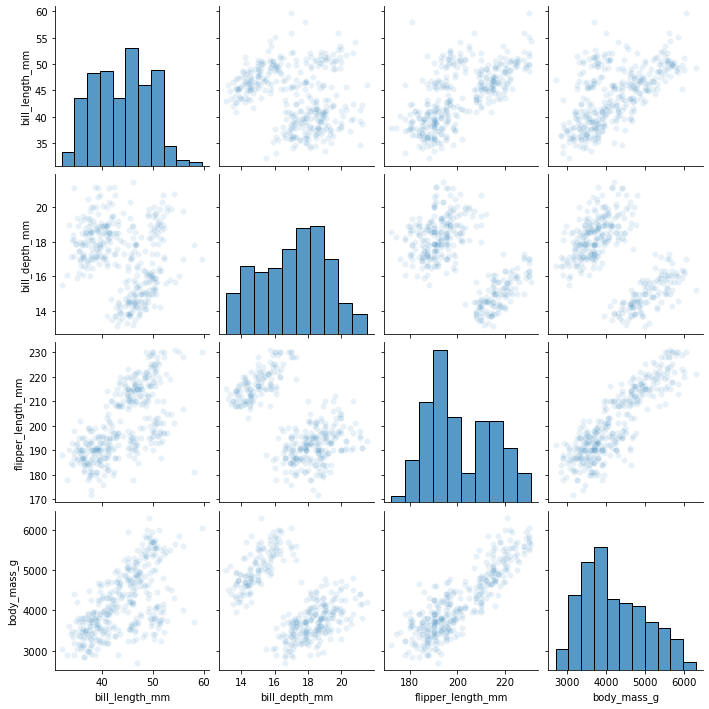
[27]:
#coloring based on a categorical variable is very natural
sns.pairplot(
my_dataset,
hue='species'
)
[27]:
<seaborn.axisgrid.PairGrid at 0x7fdd6f1e9760>
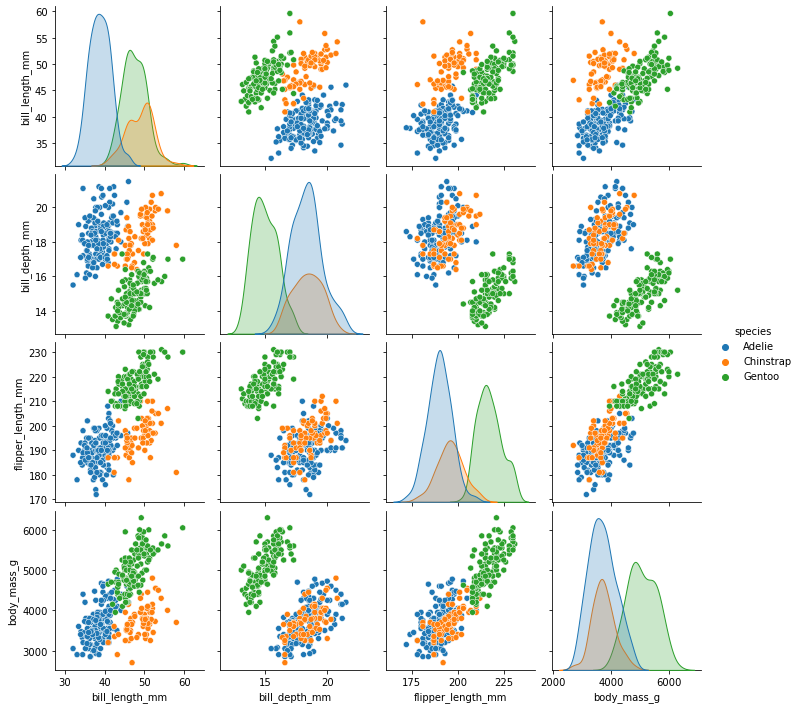
Our histograms have been transformed into (normalized?) densities.
There are way more types of plots where that came from
[28]:
sns.violinplot(
x=my_dataset.bill_depth_mm
)
[28]:
<AxesSubplot:xlabel='bill_depth_mm'>
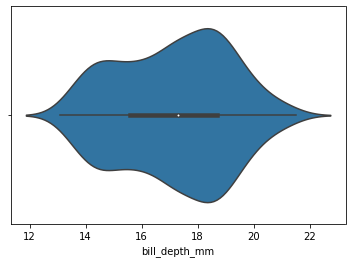
[29]:
sns.stripplot(
x='species',
y='bill_depth_mm',
data=my_dataset
)
[29]:
<AxesSubplot:xlabel='species', ylabel='bill_depth_mm'>
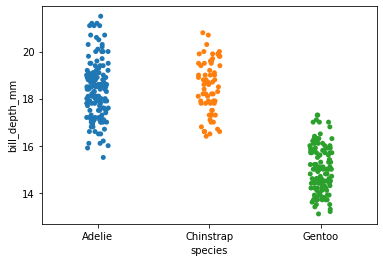
[ ]:
[ ]:
day_1_file_parsed=pd.read_csv('./Day1CountryInfo2018.txt')